Estimated read time: 9-10 minutes
This archived news story is available only for your personal, non-commercial use. Information in the story may be outdated or superseded by additional information. Reading or replaying the story in its archived form does not constitute a republication of the story.
BOSTON — Ever wonder why basketball coaches still use whiteboards?
From every level from Jr. Jazz up through the Utah Jazz, coaches are still drawing plays on their old, trusty whiteboards. But one group of data scientists is trying to change that, thanks to their new app that allows coaches to draw up plays on a tablet.
That part's been done before. But here's the new wrinkle: as the play is drawn up, the app animates a team defense's response to the action. In other words, as you draw a play up, you can see in real time how it's likely to work.
The project, called "Bhostgusters" for the "ghosts" of players it creates, is just one of dozens of research projects being presented at the Sloan Sports Analytics Conference in Boston this weekend.
Paper authors Thomas Seidl, Aditya Cherukumudi, Andrew Hartnett, Peter Carr, and Patrick Lucey explain that they use recurrent neural networks, a form of machine learning, armed with player tracking data from all 1,230 games of the 2016-17 NBA season. The goal: to effectively model how real NBA defenses respond to various offensive actions.
The ghosts even do different things depending on which defense they're modeling. The scientists have different models for elite defensive teams like San Antonio and Golden State, an average defensive team like Dallas, and a poor one like the Los Angeles Lakers.
Players going over screens, under screens, trapping, switching, hedging, helping, and closing out on shooters are all represented accurately by the modeling. Even things like player fatigue can be taken into account.
While the app's primary feature allows users to draw up a play and get tracking data for the offense and defense, it can also do the reverse: it can take tracking data and draw up the play's whiteboard diagram. From there, coaches can edit the play to see how it could have played out if a different pass were made or a different screen set.
But Bhostgusters isn't the only intriguing project being showed off at SSAC. Here's more of what's going on at the conference:
Who's really a good shooter?
One of the biggest problems in basketball analysis is trying to figure out which good shooting performances are real, and which are just a so-so shooter getting lucky. Take the Utah Jazz's list of 3-point percentage leaders, for example.
1. Joe Ingles: 45.3 percent
2. Jonas Jerebko: 43.5 percent
3. Raul Neto: 41.9 percent
4. Royce O'Neale: 39.8 percent
Joe Ingles has been a good shooter for his entire career, so we can pretty safely say his performance is pretty real. But Jerebko shot just 34.6 percent last year, Neto 32.3 percent, and O'Neale 35.6 percent in his last season in Europe. Have these players gotten better from behind the arc, or are they just getting more of their shots to bounce in right now, but will see a regression to the mean soon?
Rachel Marty tries to address that problem in her research, entitled "High-resolution shot capture reveals systematic biases and an improved method for shooter evaluation." In her paper, she uses data from 22 million shots across all levels of basketball from the "Noahlytics" system, which tracks at high-resolution how each shot taken hits the cylinder as it goes through.
The system gives each shot an x and a y coordinate for where the shot hits the basket (with the origin in the center of the hoop) and adds the angle the shot lands on the rim at.
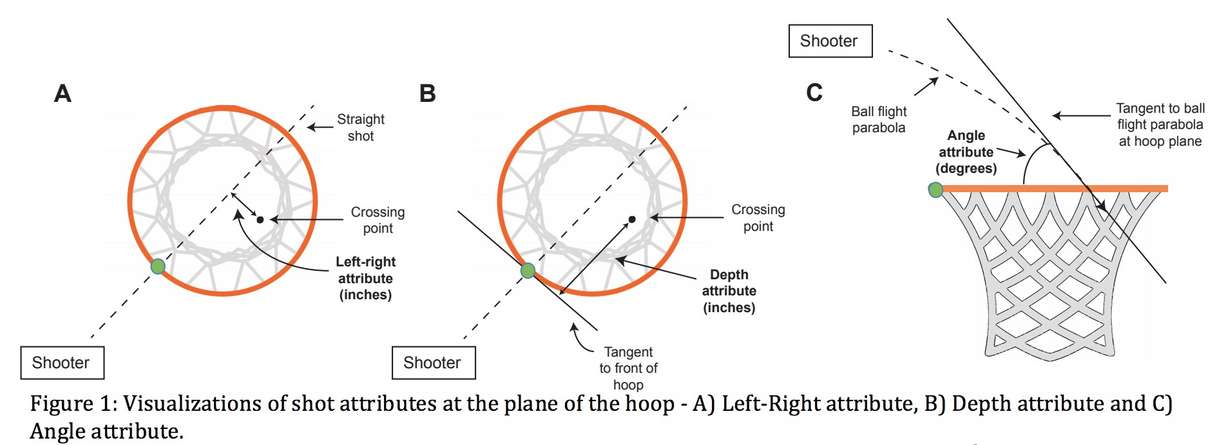
Then, she takes a look at how players tend to shoot depending on where they take their shots from. Do most players tend to miss to the left or right?
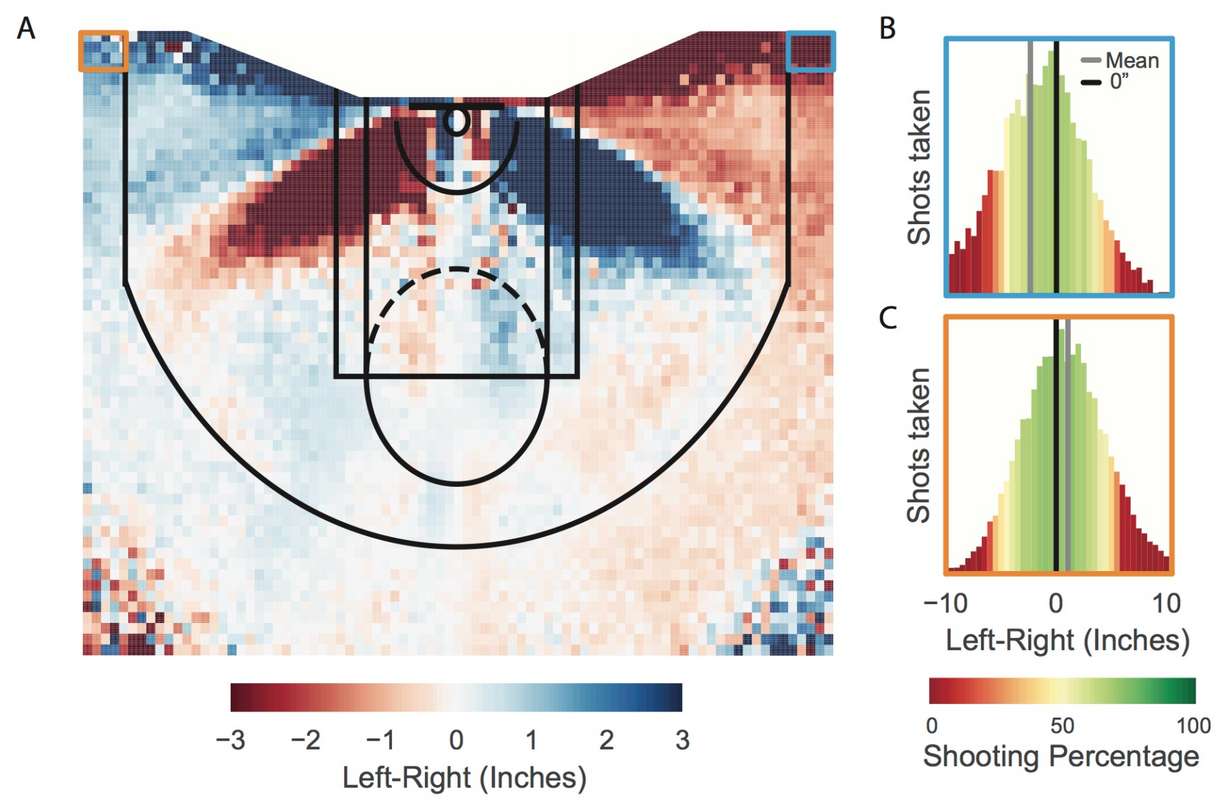
Besides the bank shots, the deeply colored zones at a 45 degree angle from the rim, everything else is pretty close to the center. The only other big location of difference is when players take baseline shots. There, their aiming is off: from the left corner, players shoot about 1.05 inches to the right of center on average, and from the right corner, players shoot about 2.34 inches to the left of the center of the hoop from their perspective.
Our best guess is that players try to avoid the embarrassing backboard miss by aiming a little bit towards the court, but that leads to some misses off the front of the iron.
Marty does the same thing with shot depth.
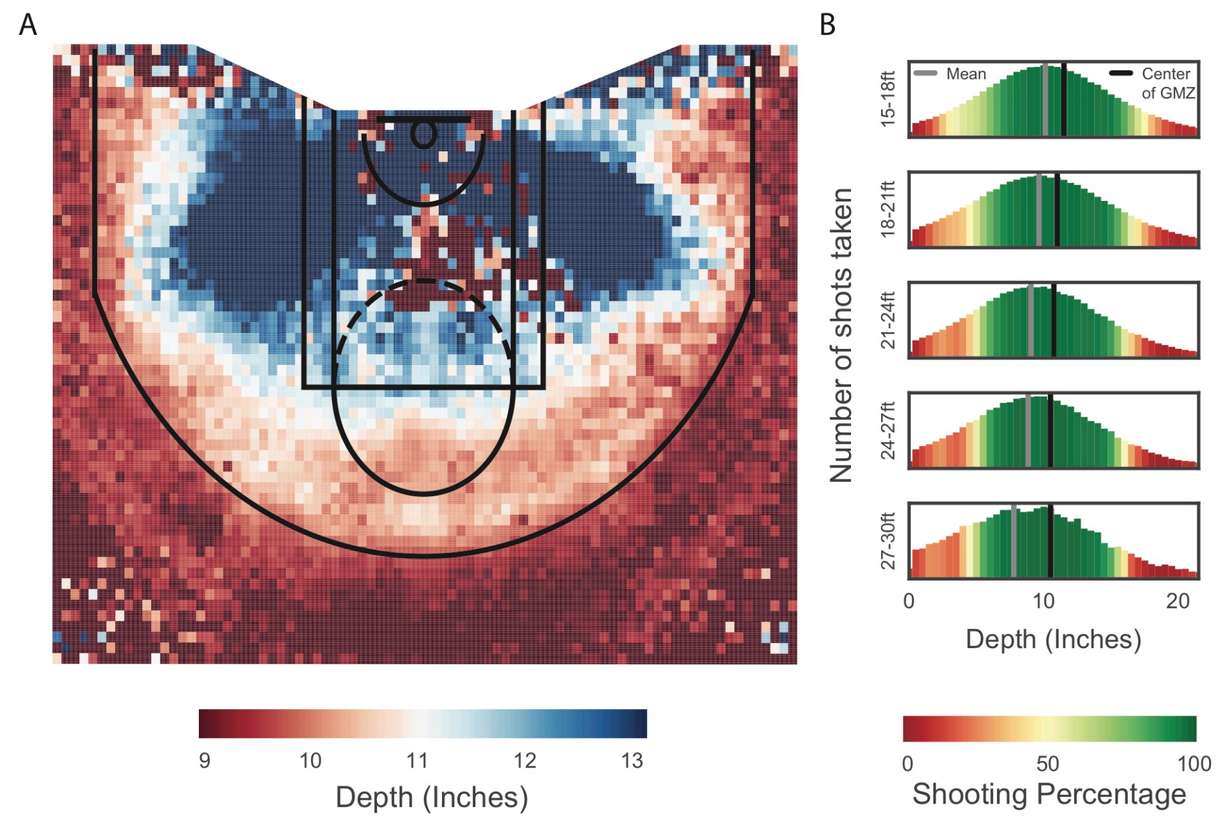
As you can see, midrange shots tend to go too long, and deep shots tend to go too short. In fact, players are missing the target by as many as three inches on average on those deep threes, certainly enough to be the difference between a miss and a make. If players consistently aimed deeper, perhaps used their legs somewhat more, they could get a higher percentage on their deep jump shots.
Finally, the paper actually goes through the work to show that having information about how a player misses shots is much more useful to determining their future shot-making success than their percentage itself. A player with a high percentage but low fundamental aim scores would be a good candidate to regress. That is, unless you can use the aim data to fix the shooter's shot to be more likely go in.
The problem with win probability
If you've read one of my postgame Triple Teams here on KSL.com, you've probably seen me use one of these graphs, from Inpredictable, modeling how a team's win probability changes throughout a game:
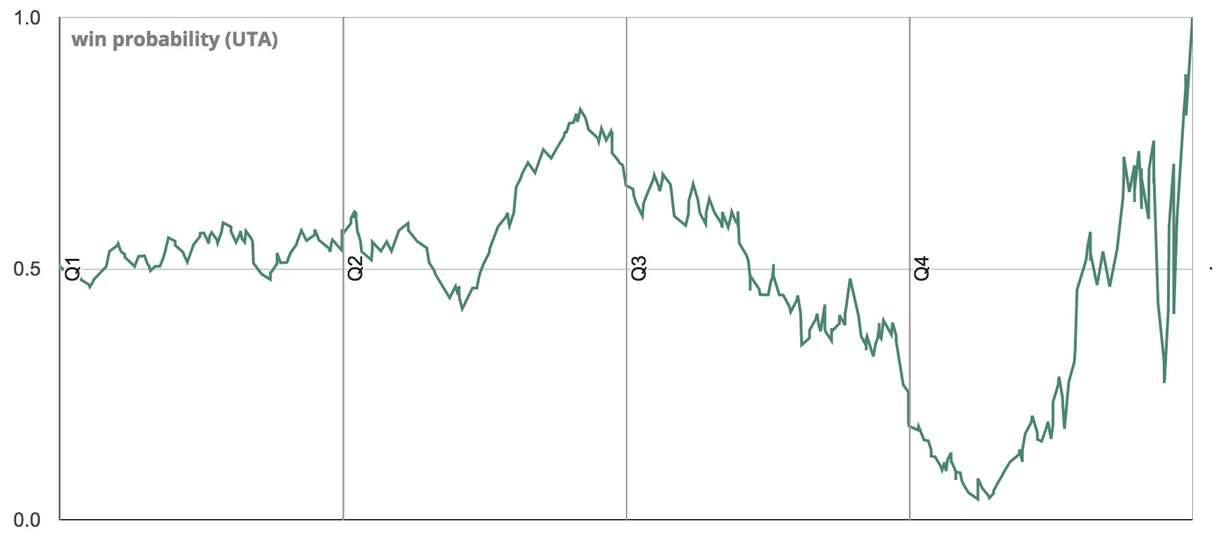
That one is from the Jazz's comeback win over the San Antonio Spurs right before the All-Star break. But you might be wondering, how does the model know what that win probability is?
Well, we don't know. While Inpredictable and ESPN have modeling systems, as do others, they've explained how they work without showing the exact calculations made. That's the problem Sujoy Ganguly and Nathan Frank of Stats, Inc. are trying to fix with their paper, The Problem with Win Probability.
Ganguly and Frank instead build a model and show their work. Their model also introduces a couple of extra quirks we haven't seen from the others: For example, one player injury can drastically impact a team's win probability for that game; this model tries its best to account for that.
Their model also outputs how sure of its prediction it is: some game states are definitely more fragile than others. Their work is a small but important step forward.
Is double teaming a good idea?
To double or not to double? That's a problem coaches often face in the NBA, especially when facing a hot or especially talented opponent. But of course, double teaming a ball-handler means that there's another player wide open. What should teams do?
Jiaxuan Wang, Ian Fox, Jonathan Skaza, Nick Linck, Satinder Singh, and Jenna Wiens from the University of Michigan tried to answer this problem in their paper. They also relied on NBA tracking data, used a neural network to detect when double teams happened in hundreds of thousands of half-court NBA possessions, and then saw how successful those possessions were. Here's the answer:
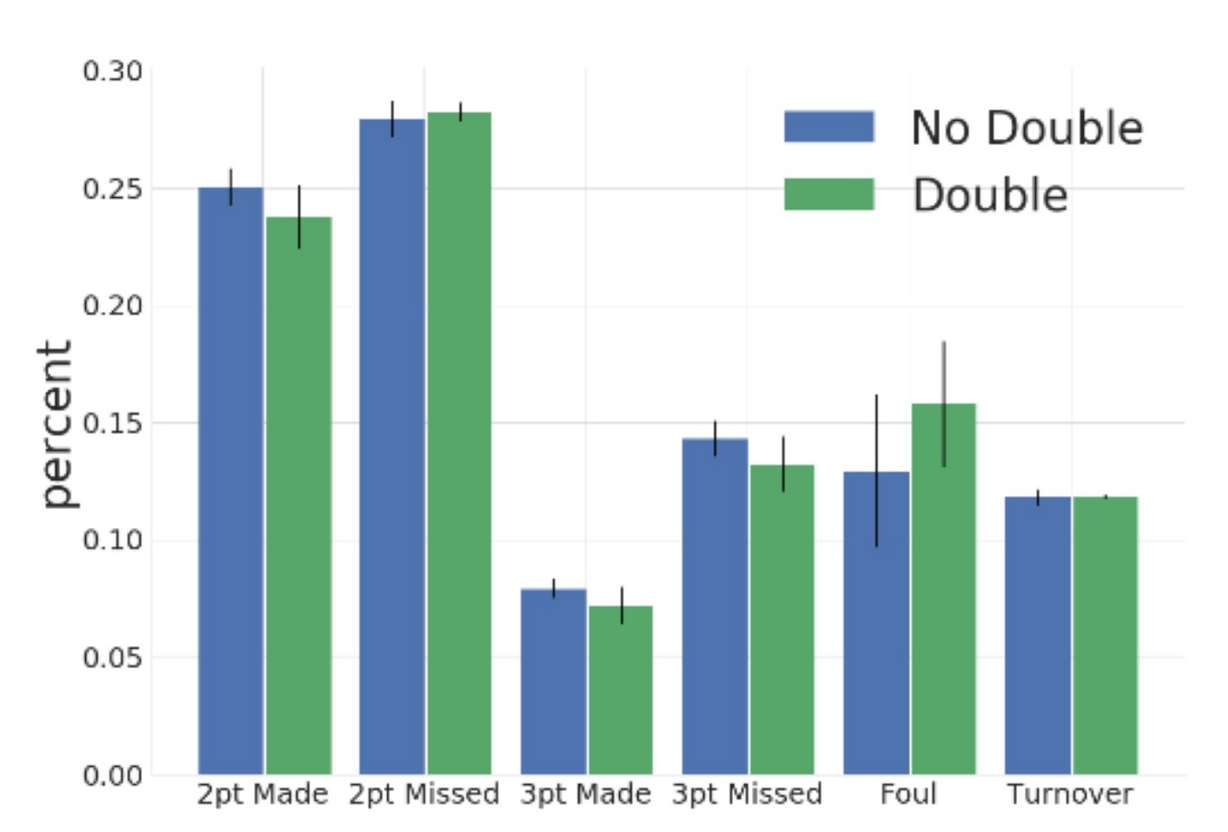
It appears that doubling makes the possession much less likely to end up in a made shot, but much more likely to end in a foul. Interestingly, turnovers stay approximately constant.
The paper makes some other conclusions about the double team as well. Passing out of a double team is much more successful than dribbling out of one, to no surprise. And it appears like it's a good idea to sometimes double team by stepping up from the paint, not necessarily always from the weak side corner.
How important is it to eliminate some bad shots?
That's not the main point of the paper "Replaying the NBA" by Nathan Sandholtz and Luke Bornn, but it is the lead tangible result. They ask the question: What if a team were to reduce contested mid-range jump shots by 20 percent while there are more than 10 seconds remaining on the shot clock? How much would that help their offensive efficiency?
Well, it depends on the team, but it looks like doing so would matter by about 0.01-0.2 points per 100 possessions. Some, but not very much at all.
But Sandholtz and Bornn's real contribution is in creating the model that leads us to be able to answer these kinds of "what if" questions with any degree of confidence.
To do so, they model every play by a Markov decision process framework, taking into account shooting percentages from all over the floor, passing, turnovers, the 24-second shot clock, and much more. It's actually a pretty impressive model, and you can imagine it being used to answer more interesting questions than the ones they pose in the paper.
What matters in the NBA draft?
Perhaps the most important task for any NBA general manager is to draft well. But how good are they actually at it? To find out, Daniel Sailofsky wrote the paper "Drafting Errors and Decision Making Bias in the NBA Draft." In it, he studied the NBA draft in a relatively straightforward manner: by collecting each season of each NCAA player drafted to the NBA between 2006 and 2013, and comparing those stats to where the player was drafted and his future NBA success.
Only one statistic examined by Sailofsky was likely to lead to both good NBA performance and a higher draft slot: assist percentage.
Meanwhile, rebounding percentage, steal percentage, free throw rate, and turnover percentage all were statistically significantly correlated with success in the NBA, but were relatively ignored in the draft evaluation process. Players with good attributes in these categories didn't seem to move up in the actual draft much at all.
On the other hand, a player's scoring totals, blocks, and height all contributed to being drafted at a higher position, but didn't have a statistically significant relationship to how those players actually played in the NBA. We've seen numerous college scorers fail in the NBA, and this study reflects that.
The study also looked at a player's age and which conference they played in. Repeatedly, players who played for big-conference schools were usually drafted higher, but only one conference was significantly correlated with NBA success: the Pac-12.
A player that was drafted younger was more likely to succeed, but only if drafted from a big conference: older players from small conferences (like Damian Lillard) also found success.
The study's methodology isn't my absolute favorite, as it eliminates players who weren't drafted (causing some survivorship bias) and measures NBA success in terms of Win Shares and Wins Produced (two good, but flawed overall stats). Still, it's an educational step forward in how far we still have to go in understanding the NBA draft.